Table of Content
These systems are built to provide relevant and personalized answers. They achieve this by using tools like natural language processing (NLP) and machine learning (ML). As a result, they can understand the context of a conversation or task.
For example, a virtual assistant with contextual AI can remember a user’s past conversations and preferences. It uses that information to give more helpful and specific responses.
As a result, these AI systems can handle many different tasks and interactions in a way that makes them seem more human.
Research indicates that contextual AI is the future. For example, Gartner reveals that search engine volumes will drop 25% by 2026 due to the increasing popularity of AI chatbots and virtual agents.
McKinsey research reveals that customer care leaders plan to allocate 23% of their gen AI investment to customer self-service and text bots. Meanwhile, 21% will be applied to customer intelligence from conversations and 18% to customer experience hyperpersonalization. These areas are where contextual AI shines.
What are the key components of Contextual AI?
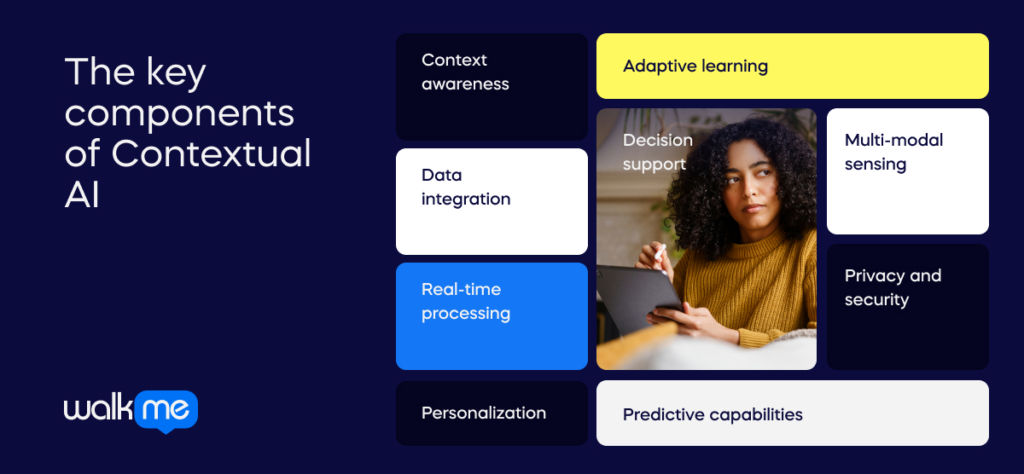
Understanding the components of contextual AI is important for understanding how it works and what makes it useful.
These features work together to ensure that the AI can adapt to different situations, give personalized answers, and make decisions based on context.
Let’s look at the components that help contextual AI work well.
Context awareness
Contextual AI needs to understand the situation it’s in. This means knowing details like user information, the environment, etc. This helps the AI give more relevant and personalized responses.
Data integration
It combines data from different sources to fully understand the situation. This data can come from sensors or social media. With a complete view, the AI can make better data-driven decisions.
Real-time processing
Contextual AI often works in real-time, so it watches and analyzes what’s happening. This allows it to quickly adjust and respond to new information.
Personalization
The AI personalises interactions by using preferences, past behaviors, and so on, making its responses more relevant to each user.
Adaptive learning
Contextual AI can learn and change over time based on new situations and user behavior. It uses tools like machine learning to improve its responses.
Decision support
It helps people and organizations make better decisions by providing insights and recommendations. This information is based on the current situation. It is a helpful tool in workplaces focusing on healthcare, finance, and business.
Predictive capabilities
Contextual AI can predict future events or trends by examining past data and the current situation. This helps determine what users might need or prefer next.
Multi-modal sensing
Contextual AI uses information from different sources like text, speech, images, and video to better understand the situation. This is important for things like self-driving cars, healthcare, and augmented reality.
Privacy and security
Because contextual AI collects and analyzes a lot of data, protecting user privacy and keeping data secure is very important. Good practices must be in place so that people can trust the AI.
Contextual AI vs contextual intelligence
Contextual AI and contextual intelligence both deal with understanding and using context. However, they do it differently.
- Contextual AI is when technology like virtual assistants or chatbots uses context to improve interactions with people.
- Contextual intelligence uses context to make better decisions in larger situations, such as for groups of people or businesses.
Let’s look further at the differences between these two terms.
Contextual AI | Contextual intelligence | |
Definition | A type of artificial intelligence that understands and responds based on the situation. For example, it looks at past conversations to provide better answers. | Using information about the situation to make decisions. It helps people and organizations make smarter choices by understanding their context. |
Focus | Aims to make technology smarter by understanding the context of data. It helps AI systems to interact better, as it can give more relevant responses. | Focuses on using context to improve decision-making for situations involving people or technology. |
Techniques | Uses tools like natural language processing (NLP) to understand human language and machine learning (ML) to learn from data. | Involves gathering and analyzing information from different sources to understand the full situation. |
Goals | To make technology interactions more useful and personalized. To improve user experience by understanding the context and providing better responses. | To enhance decision-making. Making smart choices and handling situations effectively. Adapting to changes by understanding context. |
Examples of use | Siri or Alexa uses contextual AI to give better answers based on past interactions. Customer support chatbots use contextual AI to offer personalized help by looking at past issues and current needs. Online stores suggest products based on previous searches and purchases. | Companies use contextual Intelligence to adjust their plans based on market trends and competitors. Doctors use it to choose the best treatments by considering a patient’s history and current symptoms. Organizations use contextual Intelligence to manage emergencies by understanding all aspects of the situation. |
Use cases for contextual AI
Contextual AI is used in the workplace to improve workflows. It helps improve customer support, recommend products online, and assist doctors in patient care.
Here are three examples of how contextual AI is used:
Customer support
When customers reach out for support, the contextual AI system analyzes their past purchases and interactions to deliver a more informed and personalized response.
By understanding the customer’s history context, the AI can decide when to escalate the issue to a higher level of support. This intelligent approach makes customer support more efficient, ensuring each response is tailored to the customer’s unique needs and situation.
E-commerce recommendations
Online stores employ contextual AI to suggest products that align with users’ past browsing and purchase history. The system carefully analyzes shopping patterns to offer more precise recommendations.
It also considers recent searches to highlight the most relevant items, making it easier for users to find products that suit their preferences. This method enhances the shopping experience by helping users discover items they will likely enjoy.
Healthcare
In healthcare, contextual AI assists doctors by analyzing patient data. It reviews a patient’s medical history alongside their current symptoms to aid in making accurate diagnoses.
This technology streamlines the diagnostic process, allowing doctors to deliver more personalized and precise care. As a result, healthcare providers can offer better treatment and tailored advice based on a comprehensive understanding of each patient’s unique health profile.
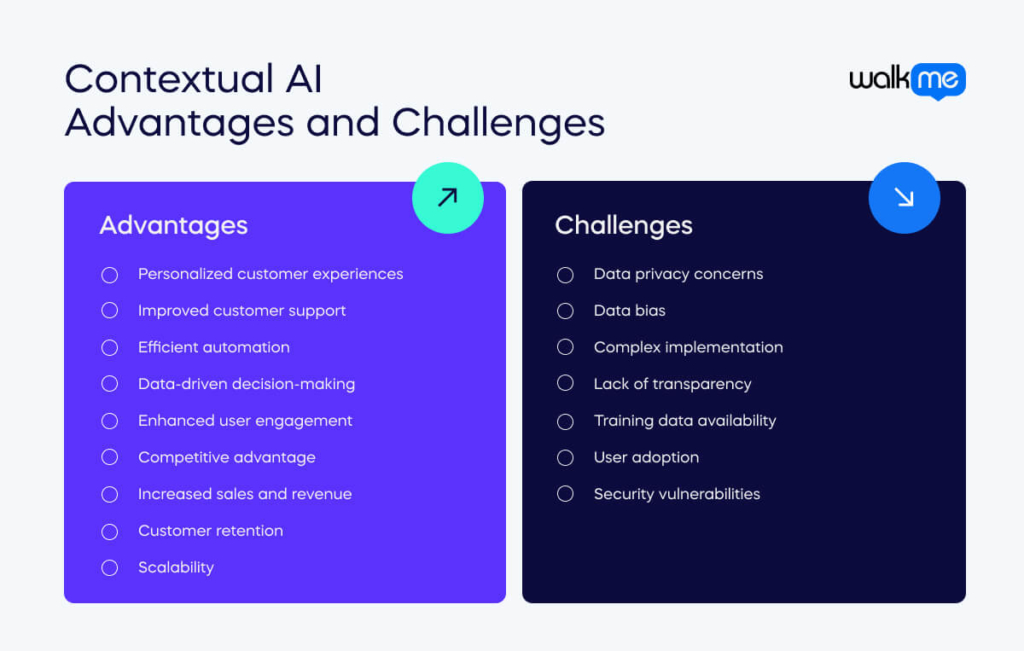
What are the advantages of contextual AI?
Using contextual AI offers many benefits. It helps companies make their services more personal and improves their operations.
As a result, businesses can offer better customer experiences, run more efficiently, and stay ahead of their competitors. Here’s how contextual AI helps:
Personalized customer experiences
Contextual AI enhances user experiences by tailoring recommendations based on individual preferences and past interactions. For instance, an e-commerce site might suggest products that align with a customer’s previous searches and purchases. This level of personalization makes interactions feel more relevant and engaging, increasing overall customer satisfaction.
Improved customer support
Contextual AI improves customer support by interpreting the context of each inquiry to provide accurate and timely responses. Instead of generic answers, the system delivers solutions considering the user’s history and current issues. This reduces the time customers spend waiting for help and minimizes the chances of errors or misunderstandings.
Efficient automation
The automation capabilities of contextual AI streamline routine tasks, such as processing orders or handling standard queries. This efficiency not only speeds up operations but also cuts down on operational costs. With AI managing repetitive tasks, employees can focus on more complex and high-value activities.
Data-driven decision-making
Contextual AI uses data analysis to support smarter business decisions. By examining patterns and trends in large datasets, AI provides valuable insights that can guide marketing and inventory management strategies. This data-driven approach helps businesses make informed decisions aligned with current market dynamics.
Enhanced user engagement
Contextual AI keeps people more engaged with products and services by delivering content tailored to users’ interests. Personalized content and recommendations make interactions more enjoyable and relevant. As a result, users are more likely to interact with and return to platforms that understand their preferences.
Competitive advantage
Adopting contextual AI gives companies a competitive edge by offering superior, customized experiences to customers. This technological advantage helps businesses stay ahead of industry trends and meet evolving customer expectations. As a result, companies can differentiate themselves from competitors and attract more customers.
Increased sales and revenue
Contextual AI boosts sales by providing targeted recommendations based on users’ browsing and purchase histories. These personalized suggestions often lead to higher conversion rates and increased revenue. Customers who receive recommendations that align with their interests are more likely to make additional purchases.
Customer retention
Contextual AI helps businesses maintain customer loyalty by delivering relevant interactions and content. The technology keeps customers engaged and satisfied by understanding and responding to individual preferences. This relevance enhances the likelihood that customers will continue to choose the brand over time.
Scalability
Contextual AI supports business growth by efficiently handling larger volumes of interactions and tasks. As a company expands, AI systems can scale to manage increased demand without requiring proportional increases in staff. This scalability ensures that businesses can grow while maintaining high levels of service.
What are the challenges of contextual AI?
Now, let’s examine the challenges of using contextual AI. While it has many benefits, it also has some difficulties. Understanding these challenges is important for using contextual AI effectively and responsibly.
Data privacy concerns
Collecting and analyzing personal data for contextual AI raises significant privacy concerns. Users may worry about how their information is used and whether it is adequately protected. Ensuring robust data security measures and transparent privacy policies is crucial to addressing these concerns and maintaining user trust.
Data bias
Contextual AI systems can inherit biases from the data they are trained on, leading to skewed or unfair outcomes. For example, the AI might make biased recommendations or decisions if the training data is not diverse. Identifying and mitigating these biases is essential for fair and equitable AI performance.
Complex implementation
Implementing contextual AI can be a complex and costly process. Businesses must invest in advanced technology and integrate it with existing systems, often requiring specialized expertise. This complexity can be a barrier for smaller organizations or those with limited resources.
Lack of transparency
Many AI systems operate in ways that are not easily understandable to users or stakeholders. This lack of transparency can make it difficult to discern how decisions are made, leading to potential trust issues. Providing clear explanations and insights into how AI systems work can help build confidence in the technology.
Training data availability
Effective contextual AI relies on access to high-quality, diverse training data. However, gathering and preparing this data can be challenging and expensive. The AI’s performance and accuracy can be compromised without sufficient and representative data.
User adoption
Some users may hesitate to embrace AI technologies due to concerns about reliability or a preference for human interaction. Overcoming this resistance requires demonstrating the value and effectiveness of AI while addressing any fears or misconceptions users may have.
Security vulnerabilities
Contextual AI systems are potential targets for cyberattacks, which can lead to data breaches and other security issues. Implementing strong security measures to protect these systems from threats and ensure that sensitive information remains secure is essential.
How can DAPs help with Contextual AI?
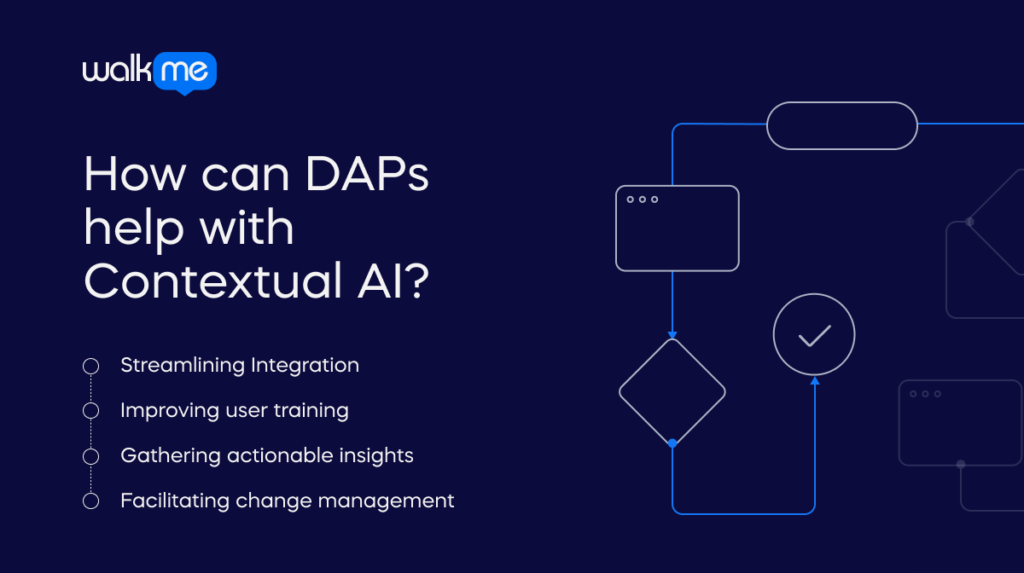
Digital adoption platforms (DAPs) can be an important part of making contextual AI more effective.
When businesses onboard a DAP, they can improve how they use this technology.
The platform provides tools that help companies to get the most out of their AI investments.
Let’s examine how DAPs contribute to making contextual AI more efficient and impactful in various business settings.
Streamlining Integration
Adding contextual AI to existing systems is easier with the help of digital adoption platforms (DAPs). These platforms provide tools to ensure the new AI works well with current processes.
Improving user training
Guided help and training are important. They help users learn to use contextual AI well. With step-by-step instructions and in-app support, users gain confidence and can make the most of the new technology.
Gathering actionable insights
DAPs can collect data on how people interact with contextual AI. This information helps to improve the way contextual AI is used, making it better suited to user needs.
Facilitating change management
Moving to using contextual AI involves solving problems, such as people not wanting to use it. Support from DAPs ensures that this change is handled smoothly and with less stress.
Last Updated: